Due to the growing realization that traditional solutions to individual global challenges often fail to address their root and interconnected causes, impact-focused organizations are increasingly embracing approaches like systems thinking, and striving to develop solutions that ultimately lead to positive systemic change. This requires viewing social issues not as isolated problems, but as part of a broader system, and exploring the interrelationships between the various components of that system in order to develop lasting solutions.
However, this has created a unique challenge when it comes to monitoring and evaluating the impact of these initiatives: It is hard enough to measure true impact amongst direct stakeholders, and doing so at a systems level magnifies this difficulty.
Navigating these challenges requires systems change-focused organizations and initiatives to adjust the questions they ask, and to employ a broader set of measurement tools that allow them to separate the signal from the noise.
The challenges of measuring systems change
Viewing individual social challenges as discrete issues may make them seem relatively straightforward, but viewing them as part of broader systems reveals these issues to be comparatively decentralized and diffuse. Understanding how any individual initiative moves the needle is deeply complex, making it difficult to measure progress. This challenge is driven by several factors:
- Multivariate complexity: Systems are built from multiple interconnected parts. With each extra variable increasing the system’s complexity, the difficulty of isolating the effects of interventions or actions on the system as a whole can rise exponentially.
- Long timelines: Changes in systems may not be immediately apparent, making it challenging to attribute observed changes to particular actions or events.
- Non-linearity: Small changes in one part of the system can lead to large, unpredictable effects in other parts. This unpredictability makes it difficult to establish clear-cut metrics or targets for measurement.
- Emergent properties: Characteristics can arise from the interactions between individual components of the system, rather than being present in the components themselves. These properties can be challenging to predict and measure.
As a result of these complexities, it’s difficult to measure an initiative’s impact on systems by the usual methods. For instance, theories of change and logframes fall short in the context of systems change work, which can be inherently non-linear, long-term and susceptible to resistance and push-back from the system. It’s clear that organizations need different measurement tools to assess the impact of systems change initiatives. But even before considering a broader set of tools, they need to be asking different questions that can help them understand the changing dynamics of a system.
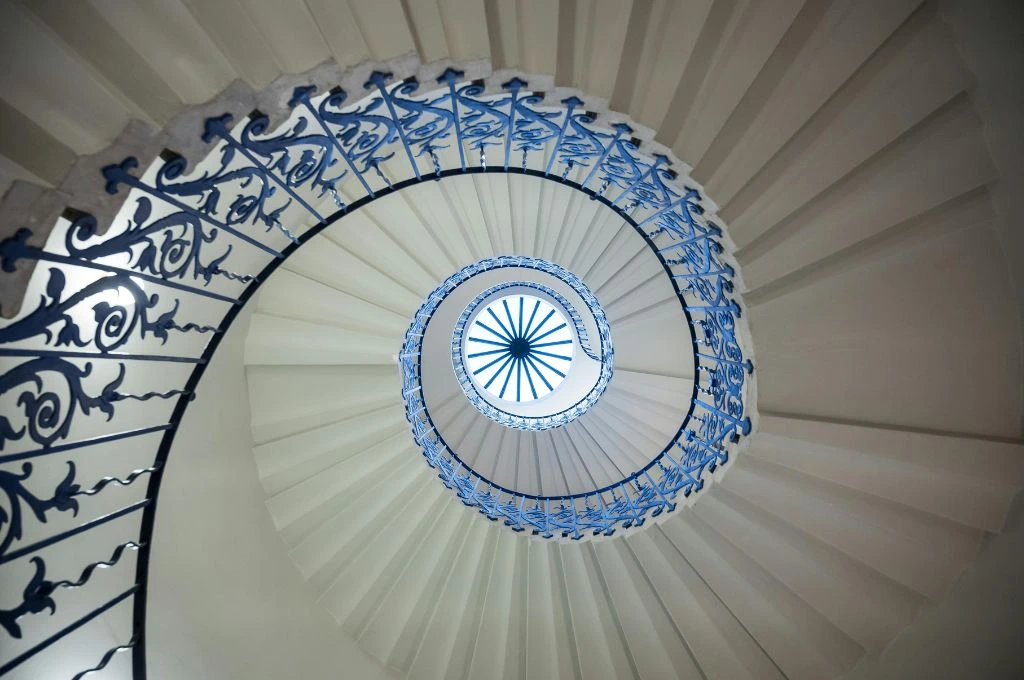
The questions organizations should be asking when measuring systems change
Measuring systems change fundamentally requires organizations to move from measurements focused on static points in time towards indicators that determine the shape a system will take over time. In particular, three shifts in measurement approaches can help them understand their impact on systems change, including:
- Measuring the rate and trajectory of impact instead of the amount of impact
- Measuring a sentiment to act instead of an action
- Measuring their influence on the most influential actors in the system, instead of their own direct influence on the system
We dig into each of these shifts below, and highlight tools that can be used to support these new approaches to measurement and analysis.
1. Measuring the rate and trajectory of impact
Ultimately, an organization or initiative’s impact on a system can only be assessed in the long term. Trying to measure impact at a single point in time is futile, but organizations can try to understand if their efforts are helping to move the system faster along its trajectory—and if they are helping to shift the trajectory itself. To that end, they need to first develop a view of the existing trajectory of the system. Is the system linear? Is it likely to exhibit exponential change over time? If systems change efforts gain traction, when can the pace of change be expected to slow down?
At Dalberg, we have found it useful to use standard market dynamics curves to help analyze these questions—including the S curve, an approach that commonly comes up in social impact work.
S-curves are often closely associated with the adoption of technology in industry. These curves illustrate that product adoption typically follows an S-shaped curve marked by a tipping point where momentum and market share surge rapidly. For example, Dalberg has used data to estimate what the S-curve looked like for solar lantern adoption before and after one of our client’s investments. We demonstrated that the highest return on investment was generated by driving the market to the tipping point faster, after which network dynamics take over (i.e., customers are much more likely to trust and buy solar lanterns once they have seen a neighbor use one). After that tipping point is reached, we found that the client could redeploy their efforts in other markets.
If an organization operates on the assumption that market dynamics will follow an S curve, it is critical for them to understand where on the curve the system was before an intervention, and at what pace it was moving. The main analytical question then switches to “How did my initiative affect the pace of change in the market?” This may sound esoteric, but in a multivariate system, the broad question of “How much of the current ecosystem’s practices are attributable to my initiative” is a lot harder for stakeholders to answer than the very specific question of “How much did my initiative increase the speed of adoption.” Given the significant statistical noise and complexity in a system, measuring your initiative’s effect on the rate of change can provide a tangible tool to understand how you are driving impact and supporting ongoing feedback loops.
2. Measuring sentiment change
It’s hard to know how much—if at all—a protest organized by Greta Thunberg (to take one hypothetical example) may lead to a policy shift in the European parliament. But thanks to technology, it is possible to understand how the sentiment of a system shifts over time—and even how it changes in direct response to an event. More broadly, when trying to ascertain whether a systems change is attributable to your action, it is often easier to ask how you are shifting the “sentiment to act.”
An important tool in this regard is the application of sentiment analysis on the large amount of user-generated text produced on digital platforms. It allows organizations to measure stakeholder perceptions of their work at scale and over time, which enables these organizations to assess the opinions of both specific stakeholders and the broader public.
To accomplish this, sentiment analysis uses machine learning techniques to extract the tone of data to detect signals of change. Common data sources include social media posts, web browser search data, and online reviews and comments. In industry, this tool can help organizations leverage the vast amounts of textual data available online, enabling them to make data-driven decisions and understand the public sentiment toward—and therefore the likely sustainability of—their programs. In systems change, this tool can allow public and stakeholder sentiment to be used as a leading indicator to help measure the initiative’s long-term progress toward changing the system.
For example, at Dalberg we have used sentiment analysis to understand a climate funder’s advocacy portfolio, tracing how their efforts created short-term sentiment shifts and how much of that sustained over time. This led to important feedback loops that enabled them to refine their current programming.
With emerging artificial intelligence models, sentiment analysis will be further super-charged, boosting our ability to process, structure and link different pieces of data for better measurement. But it’s important to recognize that this technology and access to data will need to have safeguards (after all, this approach is not dissimilar to how social media platforms have been used to understand and then manipulate user behavior).
3. Measuring the influence of actors in the system
Most organizations overestimate their influence on a system. Our prehistoric brains are designed to understand social interactions among village-sized groups of people, and we often struggle to process the fact that any system has millions of conversations happening concurrently, reflecting a vast diversity of views. But it is also true that in any system there are a small minority of influential nodes that shape the thinking of the majority. Understanding your influence on the most influential of these nodes is an efficient way to understand, to some degree, how much any changes to the system can be attributed to your actions.
To that end, a “power map” is a valuable tool for assessing influence within a system, focusing on the specific actors you are trying to influence. While a general stakeholder or actor map helps you understand who is directly and indirectly impacted by a system, a power map looks at the strength of these actors’ relationships, and can help you identify the 20% of actors who are driving 80% of the influence. For program evaluators, understanding an initiative’s impact on these influential nodes makes an otherwise overwhelming macro analysis a lot more manageable.
In some instances, this analysis can be about events and not people. For example, an organization might ask whether its point of view was represented in the room when a major decision was made. We used that approach at Dalberg, when we were asked to look at how the financial inclusion ecosystem had been shaped over the last two decades by a specific actor that had attempted to influence its trajectory. That’s a big question that would be nearly impossible to answer if we approached it broadly, but it’s possible to break that question into specific components that, when taken together, can provide some clarity. For instance, we could assess whether the client influenced the Kenyan ecosystem when M-PESA, the first at-scale mobile money product, was launched. We could ask whether it shaped the creation of the “India stack,” a digital public infrastructure that has been transformational for financial inclusion in that country. Or we could determine whether it provided inspiration for the fast-growing Chinese fintech ecosystem. Sometimes understanding systems influence can boil down to understanding influence on important events. Power maps provide a great starting point for understanding these key points of leverage.
The technological future of systems change measurement
Promising as the above tools are, our ability to measure impact at the system level is poised to take giant leaps forward in the coming years, as technology continues to develop. For instance, sensing technology is proliferating and coming down in price, as are the GSM chips that can be inserted into products to transmit usage data. Fast-moving low-earth satellites have revolutionized geospatial data costs and fidelity. Combined with the proliferation of digital data on the internet (including from mobile devices), the huge increases in processing power—and of course the incredible sense-making capabilities of new AI technologies—our capacity to measure the impact of systems change initiatives is primed for transformative growth.
As systems change efforts become increasingly common, a robust performance management approach will be necessary—one that transcends static output and outcome metrics, delving into questions of momentum, influence and scale. In this article, we have provided a starting point for how these efforts can be developed, but watch this space.
This article was originally published on Next Billion.